your current location is:Home > carHomecar
Tesla robot only sells for more than 100,000, FSD pushes 160,000 owners to test
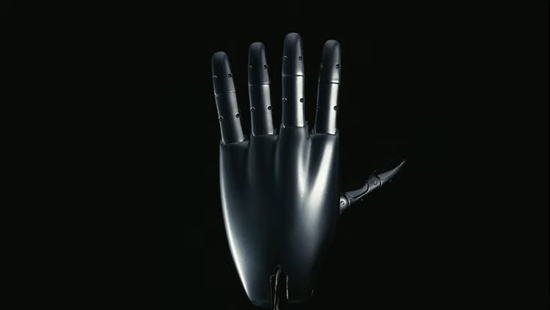
Musk: Eliminating poverty is up to me.
"Tesla robots will sell for $20,000 (equivalent to 140,000 yuan) in the future, much lower than the price of Tesla cars."
"With the Tesla robot, poverty can be eradicated, and humans can freely choose their careers."
This is on Tesla AI DAY this year. While taking out a humanoid robot, Musk described to the outside world a beautiful future world in which humans and robots coexist in harmony.
FSD fully autonomous driving and the latest progress of Tesla's self-developed supercomputing Dojo are the other two major themes of this year's AI DAY.
Of course, this AI DAY still does not change the attributes of the previous "recruitment conference", and Musk's recruitment order runs from the beginning to the end.
In short, all the dry goods and unfilled pits Tesla has made in the AI field this year are fully displayed on AI DAY.
Cyber Cars will take you to the end of the article.
01
Humanoid robot walks out of PPT
The Tesla robot Tesla Bot, which appeared as the finale of the last AI DAY, was the first to debut this year, and it is the following one:
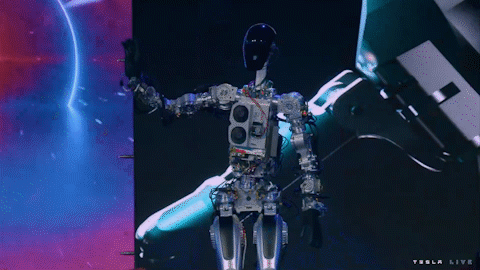
Ah, no, the one above is the verification machine, and the one below is the real owner, the Tesla Bot generation:
The prototype unveiled this time is already somewhat similar to the robot on the PPT, but the design of the torso is more mechanical.
At the same time, it can also be seen that the Tesla Bot prototype exhibited this time has the ability to move autonomously, and the flexibility of its limbs is also good. It can still be guaranteed to do a simple dance move:
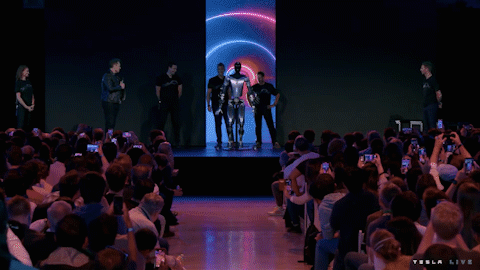
Of course, if it’s just a simple activity, it’s not too Musky. This time on AI DAY, Tesla Bot will announce more and more detailed technical parameters at the same time:
The first is the weight. The Tesla Bot first-generation prototype weighs 73 kilograms, an increase of 17 kilograms compared with the original official data.
The body flexibility data is also good. The degree of freedom of the Tesla Bot body can exceed 200, and the degree of freedom of the palm can reach 27.
Musk said that the robot on display can already achieve the ability to move autonomously, operate tools, and do some simple and repetitive tasks, while the palm can also carry 20 pounds (about 9 kilograms) of heavy objects.
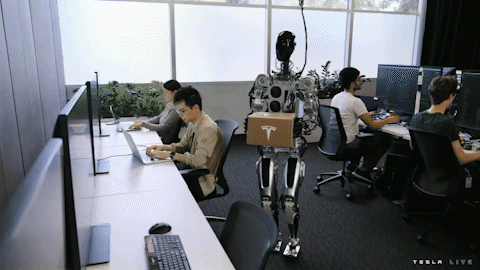
In terms of body layout, most of Tesla Bot's actuators are concentrated in the limbs. The power battery system is arranged on the torso, equipped with a 2.3kWh battery pack, with a nominal voltage output of 52V.
The computing hardware is also located in the torso part. The Tesla Bot is equipped with an FSD chip of the same Tesla model, which supports Wi-Fi and 4G (LTE) network transmission.
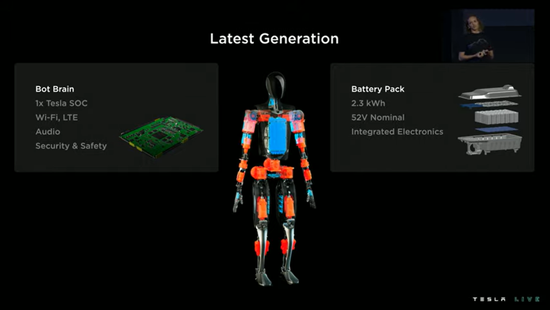
In addition, Tesla Bot also embeds Tesla's technology in autonomous driving, including software algorithms and pure vision solutions of the FSD autopilot system.
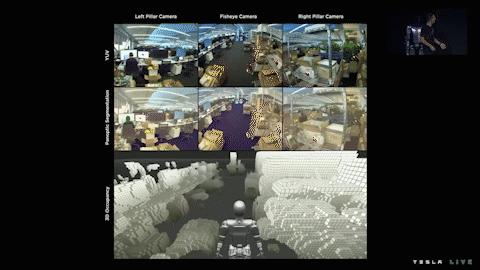
In addition to the above parameters, more R&D process and technical parameters behind Tesla Bot are also disclosed:
In terms of safety performance, Tesla Bot applies some of the technologies of Tesla's car crash experiment to ensure that the robot will not suffer major damage when it falls or is hit (the battery pack is on the chest, and it is not good for a street robot to spontaneously ignite).
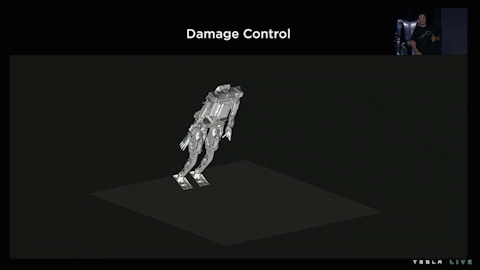
At the same time, considering the Tesla Bot's own weight and use, the flexibility and pressure-bearing capacity of the joints also need to be considered. In this regard, the Tesla Bot's joint design references human skeletal tissue.
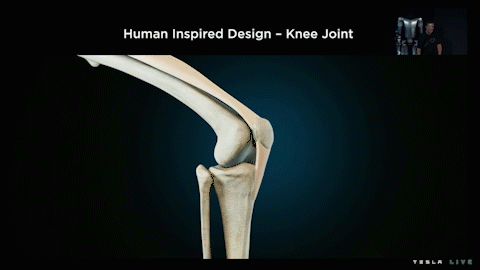
In order to ensure the pressure and flexibility of the 28 joint actuators of the whole body in different states, while taking into account efficiency and energy consumption, Tesla simulated through point clouds and designed 6 actuators with different torque outputs to undertake mobile tasks .
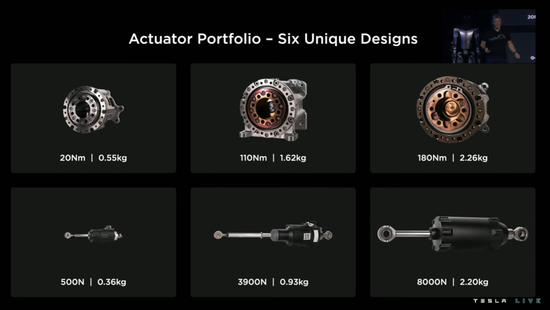
In terms of software details, Tesla mainly emphasizes the following parts:
First of all, as a humanoid robot, it needs to face various risks in the physical world, so Tesla officials emphasize that Tesla Bot needs to have a sense of autonomy at the physical level, such as an understanding of its own torso and the real world.
Secondly, it is dynamic stability. Tesla has achieved walking balance and path planning through a lot of simulation training. After adding a navigation system, Tesla Bot can move and work autonomously, and can even find a charging station to replenish energy.
Finally, the action details of Tesla Bot are anthropomorphic. Tesla captures human movements and then visualizes them, analyzes the position of the torso, and maps them to Tesla Bot.
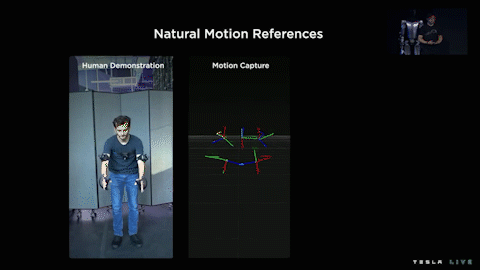
After talking about the technical details of Tesla Bot, let's try the cake painted by Musk (I respect Musk as the first person to paint the cake).
Musk said that Tesla Bot will be more expensive at the beginning, but with the increase of production, the price will also drop significantly, and it is expected that the production of millions will be reached in the future. In the end, Tesla Bot will be priced lower than Tesla's electric car, which is expected to reach $20,000 (equivalent to about 140,000 yuan).
How about a robot worth $20,000? I can't buy it, I can't buy it, I can't be fooled~
At the same time, Musk also said that with robots, the economic situation will be better and poverty will be eliminated. Because robots can replace cheap repetitive labor, people in the future will be able to choose the jobs they like.
Does this picture sound a little familiar?
but! Don't worry, the picture described by Musk, and most of the technical details, including navigation, flexibility, etc., still need a lot of refinement.
As for when it will be realized, Tesla officials said that it may be a few weeks, it may be a year, it may be a few years...
Yes, Musk has dug a hole again, Tesla Bot, after all, cannot escape the same fate as FSD.
But you can still look forward to it here. After all, most of Musk's promises came in the form of "albeit late". (You can add a dog head here)
02
FSD is still hard to get to Beta
There aren't many surprises in this part of the FSD. The production version of FSD is still far away, and the expected Beta v11 version has not arrived.
However, from the technical introduction, Tesla has already started to focus on the long tail problem (Corner Case), and the autopilot team has interpreted the technology stack in detail, as if to say, you see, autopilot is so difficult to achieve, you can't blame me bouncing.
Through this AI Day sharing, we can see that Tesla has built an autonomous driving technology stack that supports the rapid growth of FSD.
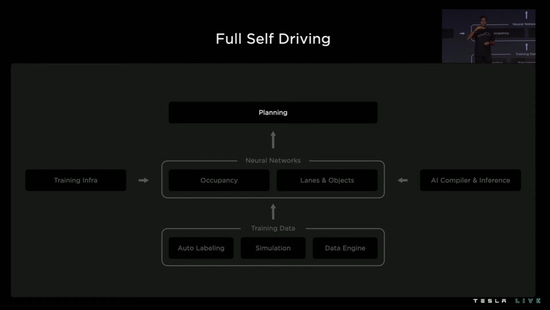
In 2021, 2,000 Tesla owners participated in the FSD Beta test, and by 2022, the number of car owners participating in the test has reached 160,000.
2022 is a very roll-out year for the Tesla Autopilot team, with 75,778 neural network models trained throughout the year, of which 281 models have achieved effective performance improvements for FSD Beta.
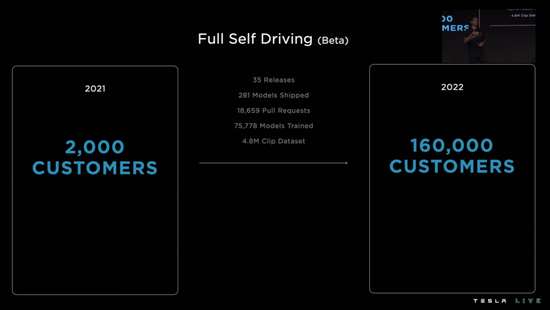
In this sharing, Tesla mainly focuses on the improvement of decision-making planning, neural network training (space occupation, lane & object detection), data training (automatic labeling, simulation, data engine) and other aspects.
Behind every autonomous driving decision is a balance based on many factors. Whether the planning choice is radical or conservative, it involves the situation of the vehicle itself, as well as other traffic participation elements, which include a lot of "relationship" processing, and different object indicators also have a lot of different states, including speed, Acceleration, static, etc. require a lot of edge computing support.
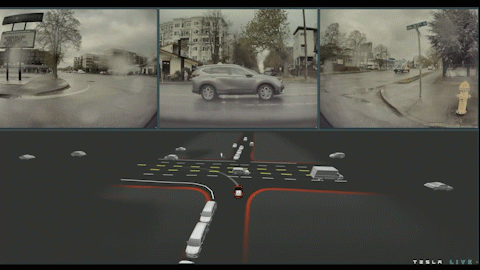
As the amount of traffic relations increases, the amount of computation will become larger and larger. In the process of a set of interaction relationships, all interaction indicators should be considered, and the most reliable solution should be calculated to form a decision tree.
At present, FSD has been able to reduce the running time of each operation to 100 microseconds, but Tesla believes that this is far from enough, and the analysis of ride comfort and external intervention factors will be added in the follow-up.
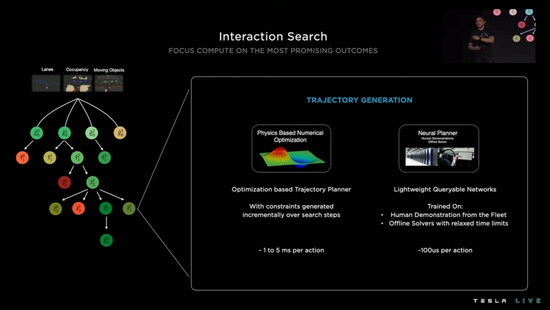
Tesla adheres to the pure visual route, but the visual solution is not perfect. Although Tesla has 8 cameras, there will always be a certain blind spot in the actual traffic scene.
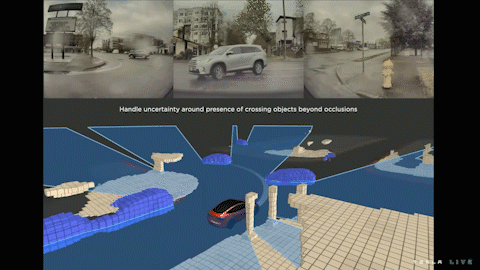
Therefore, in the neural network system of FSD, a space occupation model is introduced. It is based on geometric semantics, through camera calibration, reducing latency, analyzing how volume occupancy takes up, and rendering into vector space to represent the real world in full 3D.
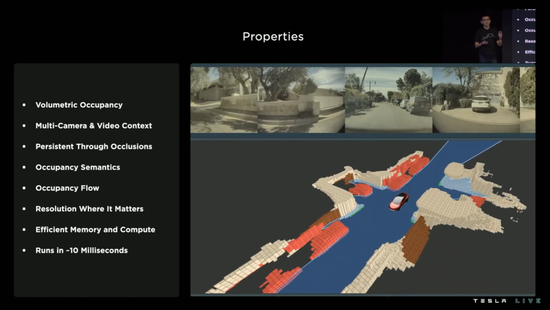
In addition, the complex road structure is also an obstacle for autonomous driving learning. Human drivers need lane lines for guidance in the real world, and the same is true for autonomous driving.
The road structure in the real world is complex and there are many connections, which greatly increases the difficulty of data processing. Tesla uses the lane detection network to convert these problems into semantics that can be recognized by computers.
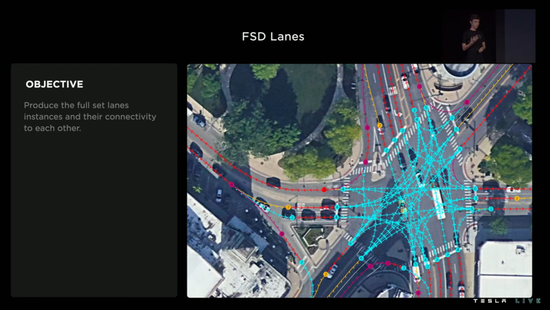
Visual perception solutions often generate a very large amount of data. The data processing compiler developed by Tesla is training video models through more efficient use of computing power, introduction of accelerators, introduction of CPUs, and reduction of bandwidth loss. Achieve a 30% increase in training speed.
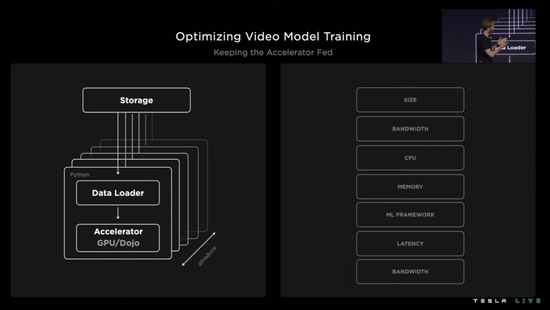
In terms of data labeling, Tesla said that data quality and labeling quality are equally important, and it is currently adopting a combination of manual labeling and automatic labeling to achieve a more detailed labeling scheme.
In terms of simulation scene construction, Tesla has also greatly improved its capabilities. The simulation scene generation is 1,000 times higher than before, and the edge geometry modeling is more refined, and it can quickly replicate the real environment, fully considering different driving scenarios.
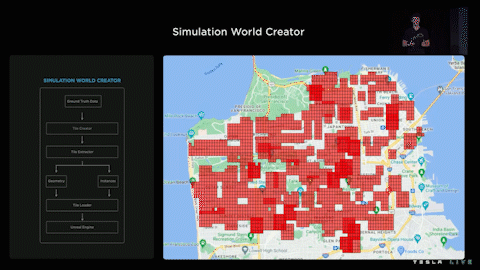
03
Why Tesla is doing supercomputing
"It is often said that as an autonomous driving company, why should Tesla develop supercomputing?"
This AI Day, Tesla gave the answer: In essence, Tesla is a hard-core technology company.
"To ask this question is to not know enough about Tesla to know what we are going to do. At Tesla, we do a lot of science and engineering related things, so there is a lot of basic data work, including reasoning, neural networks, etc., Of course it also includes supercomputing.”
After all, computing power can be said to be the basic food for training.
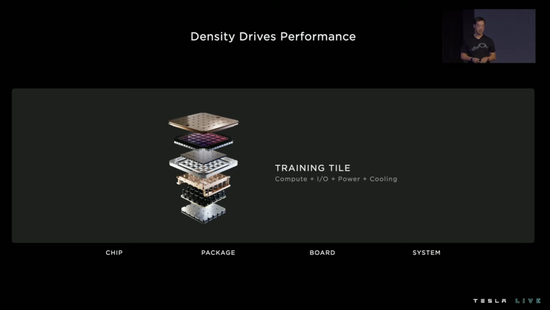
When initially designing the Dojo supercomputer, Tesla hoped to achieve substantial improvements, such as reducing the latency of self-driving training. To this end, it has carried out a series of research and development, including high-efficiency chip D1 and so on.
The D1 chip was unveiled on AI Day last year. It is a neural network training chip independently developed by Tesla. It is equipped with 50 billion transistors on a chip area of 645mm² and has a thermal design power consumption (TDP) of 400W. The calculation under FP32 precision The peak force is 22.6 TFLOPS.
The performance parameters are better than the Nvidia A100 Tensor Core GPU currently used in Tesla supercomputers. The latter chip area is 826mm², the number of transistors is 54.2 billion, TDP400W, and the peak computing power of FP32 is 19.5TFLOPS.
A single training module of the Dojo supercomputer consists of 25 D1 chips. It is reported that Tesla will launch the Dojo cabinet in the first quarter of 2023. At that time, existing supercomputers based on Nvidia A100 chips may be replaced.
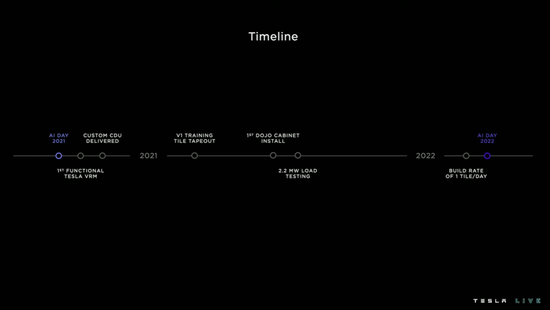
In the future, data from more than 1 million Tesla vehicles around the world will be gathered in Dojo, through which the deep neural network will be trained to help Tesla's Autopilot continue to evolve, and ultimately achieve fully autonomous driving (FSD) based on pure vision. .
Tesla said that the new Dojo supercomputer has the advantages of expanding bandwidth, reducing latency, and saving costs while having super high computing power for artificial intelligence training.
The Dojo team claims that the machine learning training computing power of a training module is enough to reach 6 "GPU computing boxes", and the cost is less than the level of "one box".
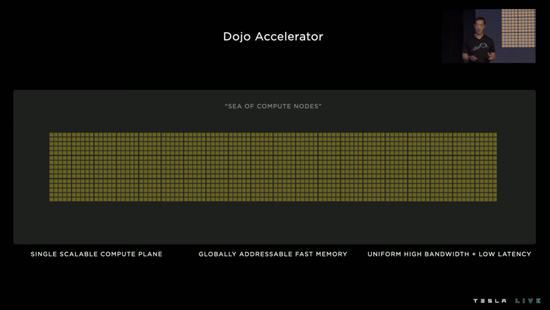
In order to achieve these performances, Tesla tried different packaging technologies and failed. In the end, Tesla abandoned the D Ram structure and adopted the S Ram structure, that is, embedded in the chip. Although the capacity was reduced, the utilization rate was significantly improved.
In addition to architectural design, considering various aspects such as virtual memory, accelerators, compilers, etc., Tesla faced various choices in the entire system design, and they also followed their own pursuit, that is, "no limit on Dojo supercomputing".
For example, in the training method, most of the selected data co-running mode is not adopted; at the data center level, a vertical integration structure is adopted, and the data center is vertically integrated.
During this process, many challenges were also encountered.
Tesla hopes to improve performance by increasing density, which poses challenges for power delivery. "We need to provide power, power to the computing chips, which will face constraints. And because the overall design is highly integrated, it needs to achieve a multi-layer vertical power solution."
Based on the above two points, Tesla builds rapid iterations, and finally reduces the CTE (coefficient of thermal expansion) by up to 50% through design and stacking.
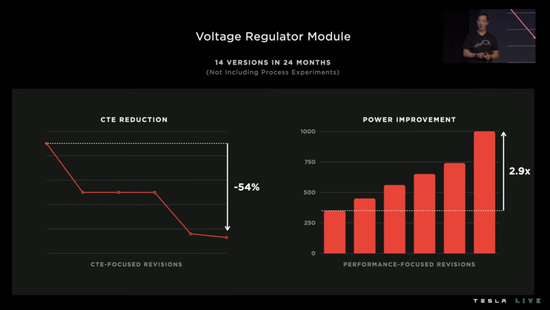
Another challenge Tesla faces: how to push the boundaries of integration.
Tesla's current power modules are x, y planes for high bandwidth communication, and everything else is stacked vertically, which involves not only the system controller, but also the loss of oscillator clock output. How to make it unaffected by the power circuit to achieve the desired degree of integration?
Tesla’s approach is a multi-pronged approach. On the one hand, it is to minimize vibration, such as by using a soft cap end, that is, the port uses a softer material to reduce vibration; on the other hand, the switching frequency is updated so that it is further away from the sensitive frequency band.
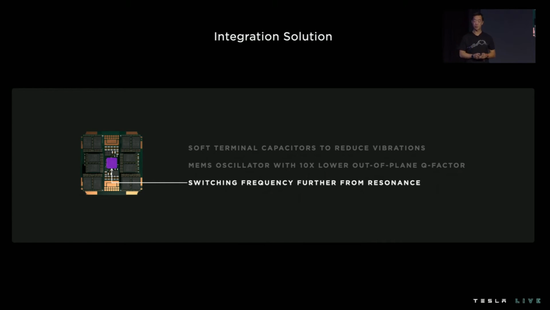
At last year's AI Day, Tesla only showed a few components of the supercomputing system, and this year it hopes to achieve more progress at the system level. Among them, the system tray is a very critical part of realizing the vision of a single accelerator, which can achieve seamless connection as a whole.
In addition, in terms of hardware, Tesla also uses high-speed Ethernet, local hardware support, etc. to accelerate the achievement of supercomputing performance; in terms of software, Tesla said that the code runs on the compiler and hardware, and it is necessary to ensure that the data is available are used jointly, so the path gradient needs to be considered in reverse.
And how to judge whether Dojo is successful and whether it has an advantage compared to the present? Tesla said to see if colleagues are willing to use it. In fact, Tesla also gave some quantitative standards, such as the workload of the system in one month, and the Dojo supercomputing can be completed in less than a week.
Of course, ultra-high computing power means huge energy consumption. In the question and answer session, Musk also said that Dojo is a supercomputer that consumes a lot of energy and requires a lot of cooling devices, so it may be provided to the market as a way of Amazon network and cloud services such as AWS.
Musk thinks it makes more sense to offer a Dojo service similar to Amazon's AWS, which he describes as an "online service that helps you train models faster for less money."
related articles
Article Comments (0)
- This article has not received comments yet, hurry up and grab the first frame~